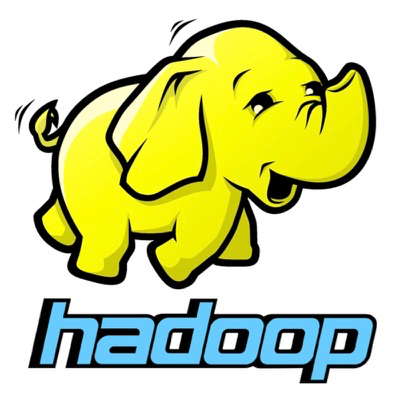
Spark MLlibの実装を読み解いてみる
こんにちは、小澤です。
今回は、Sparkの機械学習ライブラリであるMLlibがどのように実装されているのかを見てみましょう。
MLlibには、mllibパッケージとmlパッケージの2つがあります。 mlのほうが新しいパッケージとなりますので、こちらに含まれるものを見ていくことにしましょう。
はじめに
今回は、Apache Sparkのソースコードを多数掲載しています。 これらのライセンスに関しては省略していますが、すべてApache License 2.0となっています。
Apache Sparkのライセンス表記に関しては、LICENSEをご覧ください。
また、今回は個々の処理の細かい実装を追っていくことを目的とするわけではなく、Pipelienを利用したMLlibの処理の流れとしてどのようになっているのかを見ていくことを目的としています。 そのため、すべてのソースの解説をするわけではありません。 ソースコードを追っていきながら、どのクラスのどのメソッドでどの処理が実装されているのかの見通しが立てれるようになるところまでを目指すことにしたいと思います。
追っていくソース
この手のソースコードを追っていくようなものは、どこから手をつけるかが難しい部分があります。 私のオススメとして、実際に動いているコードの関数呼び出しなどから追っていくのがやりやすいです。
そのため、今回はsparkのexampleにあるPipelineExampleを始点にして見ていくことにします。
流れとしては
- Tokenizer, HashingTF, LogisticRegressionとPipelineの生成
- modelのfit
- transformでの予測
の順で見ていくことにします。
Tokenizer, HashingTF, LogisticRegressionとPipelineの生成
example中の必要なインスタンスを生成している部分を見ていきます。
// Configure an ML pipeline, which consists of three stages: tokenizer, hashingTF, and lr. val tokenizer = new Tokenizer() .setInputCol("text") .setOutputCol("words") val hashingTF = new HashingTF() .setNumFeatures(1000) .setInputCol(tokenizer.getOutputCol) .setOutputCol("features") val lr = new LogisticRegression() .setMaxIter(10) .setRegParam(0.001) val pipeline = new Pipeline() .setStages(Array(tokenizer, hashingTF, lr))
Toknizer
最初にTokenizerを見ていきます。 Tokenizerはいろいろextendsされてたりします。 その継承関係は以下のようになっています。
だいたいは、名前からおおよそ何をするためのものか想像しやすいですね。 Params, HasInputCol, HasOutputColはtraitになっています。
では、Tokenizerの実際の中身を追ってみましょう。
まずは最初にnewしているのでコンストラクタが呼び出されます。
@Since("1.2.0") class Tokenizer @Since("1.4.0") (@Since("1.4.0") override val uid: String) extends UnaryTransformer[String, Seq[String], Tokenizer] with DefaultParamsWritable { @Since("1.2.0") def this() = this(Identifiable.randomUID("tok"))
コンストラクタではuidを与えているのみとなります。 Identifiable.randomUIDについての詳細は追いませんがUUIDを取得して、一意なIDを求めています。
その次に、setInputCol, setOutputColメソッドを呼び出しています。 それぞれ、UnaryTransformerに実装されています。
/** @group setParam */ def setInputCol(value: String): T = set(inputCol, value).asInstanceOf[T] /** @group setParam */ def setOutputCol(value: String): T = set(outputCol, value).asInstanceOf[T]
setはParamsで実装されています。
/** Internal param map for user-supplied values. */ private val paramMap: ParamMap = ParamMap.empty /** * Sets a parameter in the embedded param map. */ final def set[T](param: Param[T], value: T): this.type = { set(param -> value) } /** * Sets a parameter (by name) in the embedded param map. */ protected final def set(param: String, value: Any): this.type = { set(getParam(param), value) } /** * Sets a parameter in the embedded param map. */ protected final def set(paramPair: ParamPair[_]): this.type = { shouldOwn(paramPair.param) paramMap.put(paramPair) this } /** Validates that the input param belongs to this instance. */ private def shouldOwn(param: Param[_]): Unit = { require(param.parent == uid && hasParam(param.name), s"Param $param does not belong to $this.") }
基本的には通常のMapなどのputと似たような動きをするものとなります。
getParamに関しては、HasInputCol, HasOutputColでそれぞれ定義されています。
/** * Trait for shared param inputCol. */ private[ml] trait HasInputCol extends Params { /** * Param for input column name. * @group param */ final val inputCol: Param[String] = new Param[String](this, "inputCol", "input column name") /** @group getParam */ final def getInputCol: String = $(inputCol) } /** * Trait for shared param outputCol (default: uid + "__output"). */ private[ml] trait HasOutputCol extends Params { /** * Param for output column name. * @group param */ final val outputCol: Param[String] = new Param[String](this, "outputCol", "output column name") setDefault(outputCol, uid + "__output") /** @group getParam */ final def getOutputCol: String = $(outputCol) }
ParamMapについての詳細は割愛しますが、mutable.Mapを内部で持っていて、それにいくつか機能を追加したものになります。 また、ここで新たにParamというクラスが登場しましたが、こちらはkey-valueの形式でパラメータを保持しているようなものになりますで、細かい部分は割愛します。
ここまでで、Tokenizerに必要な入出力の設定が行えました。
Tokenizerの設定についてまとめると、
- traitで定義された、inputCol, outputColという2つのパラメータを持っている
- 値をセットすることで、実際の値が入ったパラメータのMapが得られる
となります。 ざっくりとこの構造をまとめた図が以下のようになります。
少々長くなってしまいましたが、やっていることとしてはそれほど複雑ではないことがわかるかと思います。
HashingTF
次にHashingTFを見ていきます。
こちらのコンストラクタは、UnaryTransformerではなく、Transformerを直接継承している以外は、Tokenizerと同じになります。
@Since("1.2.0") class HashingTF @Since("1.4.0") (@Since("1.4.0") override val uid: String) extends Transformer with HasInputCol with HasOutputCol with DefaultParamsWritable { @Since("1.2.0") def this() = this(Identifiable.randomUID("hashingTF"))
Transformerでは、変換処理を行うための最低限の機能のみとなっています。 そのため、先ほどのTokenizerではUnaryTransformerで定義されていたようなメソッドがこのHashingTFでは直接実装されています。 この辺りの実装方法は基本的に、Tokenizerと同じになりますで、省略します。
HashingTF独自で持っている設定値としてnumFeaturesとbinaryがあります。 numFeaturesは文字列をハッシュ化する際の特徴次元になります。 binaryはfalseの場合、単語の出現回数、trueの場合は出現したか否かの0/1になります。
LogisticRegression
Pipelineに入れるものの最後は、LogisticRegressionとなります。
少々複雑ですが、基本的な流ればこれまでと変わりません。 また、「いっぱい」となっている部分は多くのParamを継承したtraitがあるため、省略しています。 ソースコードを見ていく際に必要に応じてどこが何を継承しているかも見ていきます。
コンストラクタで行っている処理はこれまでと同様ユニークなIDを設定しているのみです。
@Since("1.2.0") class LogisticRegression @Since("1.2.0") ( @Since("1.4.0") override val uid: String) extends ProbabilisticClassifier[Vector, LogisticRegression, LogisticRegressionModel] with LogisticRegressionParams with DefaultParamsWritable with Logging { @Since("1.4.0") def this() = this(Identifiable.randomUID("logreg"))
パラメータの設定は、setMaxIter, setRegParamの2つです。
この2つはLogisticRegressionParamsがextendsしている、HasMaxIter, HasRegParamで定義されているものになります。 具体的な定義の仕方は、これまで見てきたものと同様になるため省略します。
private[classification] trait LogisticRegressionParams extends ProbabilisticClassifierParams with HasRegParam with HasElasticNetParam with HasMaxIter with HasFitIntercept with HasTol with HasStandardization with HasWeightCol with HasThreshold with HasAggregationDepth {
なお、regParamの"reg"となっている部分はLogisticRegressionのRegressionとは関係なく、regularization(正則化)のパラメータなので、ご注意ください(え?誰もそんな勘違いしないって?)。
LogisticRegressionの初期化部分については、これで完了しています。
Pipeline
さて、初期化部分の最後として、Pipelineの生成を見てみましょう。 とはいえ、実はPipelineについても、初期化部分に関してはこれまでと同様です。
@Since("1.2.0") class Pipeline @Since("1.4.0") ( @Since("1.4.0") override val uid: String) extends Estimator[PipelineModel] with MLWritable { @Since("1.4.0") def this() = this(Identifiable.randomUID("pipeline"))
ユニークなIDをふっているだけですね。 Estimatorをextendsしてますが、こちらもその先がどうなっているのかは、LogisticRegressionと同様になります。
setStagesに関しても同様、パラメータの設定と同じ要領で設定しています。
/** * param for pipeline stages * @group param */ @Since("1.2.0") val stages: Param[Array[PipelineStage]] = new Param(this, "stages", "stages of the pipeline") /** @group setParam */ @Since("1.2.0") def setStages(value: Array[_ <: PipelineStage]): this.type = { set(stages, value.asInstanceOf[Array[PipelineStage]]) this }
modelのfit
さて、ここまでは機械学習を行う準備段階です。 ここからいよいよ、Pipeline内の処理を実行していくことになります。
val model = pipeline.fit(training)
とはいえ、実行しているコードだけをみれば1行ですね。 fitの中身を見てみましょう。
override def fit(dataset: Dataset[_]): PipelineModel = { transformSchema(dataset.schema, logging = true) val theStages = $(stages) // Search for the last estimator. var indexOfLastEstimator = -1 theStages.view.zipWithIndex.foreach { case (stage, index) => stage match { case _: Estimator[_] => indexOfLastEstimator = index case _ => } } var curDataset = dataset val transformers = ListBuffer.empty[Transformer] theStages.view.zipWithIndex.foreach { case (stage, index) => if (index <= indexOfLastEstimator) { val transformer = stage match { case estimator: Estimator[_] => estimator.fit(curDataset) case t: Transformer => t case _ => throw new IllegalArgumentException( s"Does not support stage $stage of type ${stage.getClass}") } if (index < indexOfLastEstimator) { curDataset = transformer.transform(curDataset) } transformers += transformer } else { transformers += stage.asInstanceOf[Transformer] } } new PipelineModel(uid, transformers.toArray).setParent(this) }
すべてを細かく見ていくことはしませんので、ざっくりと見ていきましょう。
まずは、
var indexOfLastEstimator = -1 theStages.view.zipWithIndex.foreach { case (stage, index) => stage match { case _: Estimator[_] => indexOfLastEstimator = index case _ => } }
でPipeline中の一番最後のEstimatorの位置を探しています。
exampleでは、LogisticRegressionがEstimatorを継承しており、それ以外の2つはTransformerを継承していました。 また、Estimatorというクラス名からもわかるかと思いますが、ここでインデックスの値として求めているのが、このPipelineでの機械学習の際す集的な結果となっています。
次に、こちらの部分です。
theStages.view.zipWithIndex.foreach { case (stage, index) => // indexOfLastEstimatorが先ほどインデックスを求めた最終的な出力となるモデル // Pipeline中のindexOfLastEstimatorに至るまでのすべての変換処理を行う if (index <= indexOfLastEstimator) { val transformer = stage match { case estimator: Estimator[_] => // Estimatorの場合、学習 -> 予測というプロセスになるので // 途中または最後のEstimatorで必要な処理の部分を実行している estimator.fit(curDataset) case t: Transformer => t case _ => throw new IllegalArgumentException( s"Does not support stage $stage of type ${stage.getClass}") } if (index < indexOfLastEstimator) { // transformの呼び出しによって、必要な変換処理をしている。 // 上のif文と違って、indexOfLastEstimatorは含まれないので、 // Pipelineの途中に含まれているEstimatorは学習した上でこの処理予測結果への変換を行っているが、 // 最終的なモデルは学習のみとなっている。 curDataset = transformer.transform(curDataset) } transformers += transformer } else { transformers += stage.asInstanceOf[Transformer] } }
こちらもプログラムとしては特に難しいことはしていないかと思います。
先ほど、求めておいた最後の機械学習アルゴリズムでの学習を行うまで、Pipeline中の各ステージの処理を実行しています。 いくつかコメントで何をしているのかを補足で記載しておきましたので、そちらも合わせて参考にしてください。
さて、ここからがいよいよメインの部分となります。 各Transformer, Estimatorのtransform, fitで具体的に行っている処理を見ていくことになります。
Tokenizer
まずは、Tonkenizerから見ていきましょう。 と、言いたいところですが、Tokenizerにはtransformメソッドが定義されていません。
そこで、継承元であるUnaryTransformerのtransformメソッドを見てみます。
override def transform(dataset: Dataset[_]): DataFrame = { transformSchema(dataset.schema, logging = true) val transformUDF = udf(this.createTransformFunc, outputDataType) dataset.withColumn($(outputCol), transformUDF(dataset($(inputCol)))) }
処理内容としては、Spark SQLのudf定義を行ってそれを実行しているようです(Dataset APIやSpark SQLに関してはここでは詳細は解説しません)。
実際に実行されているのはcreateTransformFuncのようなので、Tokenizerはそちらを確認してみましょう。
override protected def createTransformFunc: String => Seq[String] = { _.toLowerCase.split("\\s") }
内容はいたって単純で、全体を小文字に統一して、空白文字で区切ったものを返す処理になっています。 単純に空白文字で区切っているだけで、ステミングやストップワード除去は行っていないこともこのソースから確認できます(ストップワード除去はStopWordsRemoverというのが別途用意されています)。
また、日本語のように分かち書き(文章を単語ごとに空白文字区切る)をしない文章を扱う場合は利用できないことも確認できます。
HashingTF
次にHashingTFを見てみましょう。 こちらはTransformが定義されています。
override def transform(dataset: Dataset[_]): DataFrame = { val outputSchema = transformSchema(dataset.schema) val hashingTF = new feature.HashingTF($(numFeatures)).setBinary($(binary)) // TODO: Make the hashingTF.transform natively in ml framework to avoid extra conversion. val t = udf { terms: Seq[_] => hashingTF.transform(terms).asML } val metadata = outputSchema($(outputCol)).metadata dataset.select(col("*"), t(col($(inputCol))).as($(outputCol), metadata)) }
HashingTFでは、内部的に"mllib"の方のHashingTFを利用しています(TODOコメントにあるようにいずれこの依存はなくなるでしょう)。 その実装は以下のようになっています。
def transform(document: Iterable[_]): Vector = { val termFrequencies = mutable.HashMap.empty[Int, Double] // binaryがtrueの場合、出現したら1、falseの場合出現回数をインクリメントしたものを返す関数 val setTF = if (binary) (i: Int) => 1.0 else (i: Int) => termFrequencies.getOrElse(i, 0.0) + 1.0 // ハッシュ値を返す関数 val hashFunc: Any => Int = getHashFunction document.foreach { term => // 単語をハッシュ化して、出現回数をインクリメントする val i = Utils.nonNegativeMod(hashFunc(term), numFeatures) termFrequencies.put(i, setTF(i)) } Vectors.sparse(numFeatures, termFrequencies.toSeq) }
処理内容に関して、コメントを記載しましたが、 流れとしては、binaryがtrueの場合、出現したら1、falseの場合出現回数をインクリメントしたものと単語のハッシュ値を返すような処理を実行しています。 ハッシュ値を返す処理についての詳細を追うのはここでは割愛しますが、ここでの処理の関して簡単に補足しておきます。
機械学習で扱うデータはDataFrame APIやDataset APIで扱うような形式になっており、データの各列のことをfeature(特徴量、素性など)と呼びます。
文章などの文字列で与えられたデータをこのfeatureの形式に落とし込む方法として、Bag of Words(BoW)と呼ばれる手法があります。 これは、全てのデータに含まれる各単語をfeatureとして、マッピングするような方法です。
例えば、
- 1: 今日はいい天気です。
- 2: 明日もいい天気の予報です。
のような2件のデータに対しては、
id | 今日 | いい | 天気 | 明日 | 予報 |
---|---|---|---|---|---|
1 | 1 | 1 | 1 | 0 | 0 |
2 | 0 | 1 | 1 | 1 | 1 |
のようになります(※ 一部省略しています)。 この時、各単語の出現回数をカウントするか、出現したか否かで0/1を与えるかの2種類が考えられます。 データの数が大量になると、全体として出現する単語数に対して各文章で出現する単語数は少ないのでほとんどの要素が0になるような行列が出来上がることがわかるかと思います。
BoWの欠点としては、例えば上記2件のデータで学習を行ったのち、以下のデータを予測したいとします。
- 昨日もいい天気でした。
この時に「昨日」という単語は学習時には存在していなかったため、対応するfeatureが存在しません。 そのため、このような値が出てくることを考慮できなかったり、スムージングなどの処理が必要になることがあります。
それに対してFeature Hashing(あるいはHashing Trick)は各単語のハッシュ値が衝突しないようなハッシュ関数を用意して、 単語をハッシュ値に変換したものをfeatureとして利用します。 これによって、そのハッシュ関数の計算式に乗っかれば学習時には出現しなかった未知の単語を利用することが可能になります。
今回利用しているHashingTFはこのFeature Hashingを利用して、Tokeizerによって分割された各単語のハッシュ値ごとの出現回数(または0/1)をfeatureとして割り当てるためのものになります。
LogisticRegression
さて、最後はLogisticRegressionです。 今回のexampleだと、LogisticRegressionは最後のEstimatorなので、Pipeline中ではfitは呼び出されますが、transformが呼び出されることはありません。
そのため、ここではfitの中身を見てみましょう。
こちらもLogisticRegressionにはfitが定義されていません。 Predictorまで遡ることでその定義が見つかります。
override def fit(dataset: Dataset[_]): M = { // This handles a few items such as schema validation. // Developers only need to implement train(). transformSchema(dataset.schema, logging = true) // Cast LabelCol to DoubleType and keep the metadata. val labelMeta = dataset.schema($(labelCol)).metadata val labelCasted = dataset.withColumn($(labelCol), col($(labelCol)).cast(DoubleType), labelMeta) // Cast WeightCol to DoubleType and keep the metadata. val casted = this match { case p: HasWeightCol => if (isDefined(p.weightCol) && $(p.weightCol).nonEmpty) { val weightMeta = dataset.schema($(p.weightCol)).metadata labelCasted.withColumn($(p.weightCol), col($(p.weightCol)).cast(DoubleType), weightMeta) } else { labelCasted } case _ => labelCasted } copyValues(train(casted).setParent(this)) }
この中でのほとんどの処理はコメントにある通り、型変換のためのものになります。 学習の処理を行っているのは一番最後のtrainの呼び出しとなりますので、今回はここだけを見てみましょう。
trainはLogisticRegressionで実装されていますが、非常に長い処理になるため、 全体の解説はせず、いくつかの処理のみを見ていきたいと思います。
最適化関数の設定を行っている部分です。 手法自体の詳細は解説しないため、内部の処理までは確認しませんが、L-BFGSなどを使っているのが確認できます。
val optimizer = if ($(elasticNetParam) == 0.0 || $(regParam) == 0.0) { if (lowerBounds != null && upperBounds != null) { new BreezeLBFGSB( BDV[Double](lowerBounds), BDV[Double](upperBounds), $(maxIter), 10, $(tol)) } else { new BreezeLBFGS[BDV[Double]]($(maxIter), 10, $(tol)) } } else { val standardizationParam = $(standardization) def regParamL1Fun = (index: Int) => { // Remove the L1 penalization on the intercept val isIntercept = $(fitIntercept) && index >= numFeatures * numCoefficientSets if (isIntercept) { 0.0 } else { if (standardizationParam) { regParamL1 } else { val featureIndex = index / numCoefficientSets // If `standardization` is false, we still standardize the data // to improve the rate of convergence; as a result, we have to // perform this reverse standardization by penalizing each component // differently to get effectively the same objective function when // the training dataset is not standardized. if (featuresStd(featureIndex) != 0.0) { regParamL1 / featuresStd(featureIndex) } else { 0.0 } } } } new BreezeOWLQN[Int, BDV[Double]]($(maxIter), 10, regParamL1Fun, $(tol)) }
初期値の設定部分もいくつかの状況に合わせて分岐しているので、二値分類のところのみを確認してみましょう
/* For binary logistic regression, when we initialize the coefficients as zeros, it will converge faster if we initialize the intercept such that it follows the distribution of the labels. {{{ P(0) = 1 / (1 + \exp(b)), and P(1) = \exp(b) / (1 + \exp(b)) }}}, hence {{{ b = \log{P(1) / P(0)} = \log{count_1 / count_0} }}} */ initialCoefWithInterceptMatrix.update(0, numFeatures, math.log(histogram(1) / histogram(0)))
やってることに関しては、コメントにほとんど記載されているので、あまり解説の必要はなさそうですね。
今回見ていく最後の部分がこちらになります。 optimaizerをループさせることでパラメータの更新が行われているのが確認できるかと思います。
val states = optimizer.iterations(new CachedDiffFunction(costFun), new BDV[Double](initialCoefWithInterceptMatrix.toArray)) /* Note that in Logistic Regression, the objective history (loss + regularization) is log-likelihood which is invariant under feature standardization. As a result, the objective history from optimizer is the same as the one in the original space. */ val arrayBuilder = mutable.ArrayBuilder.make[Double] var state: optimizer.State = null while (states.hasNext) { state = states.next() arrayBuilder += state.adjustedValue }
他にも、train内では様々処理を行っています。 400行くらいある関数のため、一度に全体を見ていくのは難しいかもしれませんが、if文による分岐も多いため、「今回の処理はどこを通っているのか」で追っていくと比較的読みやすいかと思います。
また、optimizerの中の処理まで追うこともしませんでした。 こちらは実際の手法がどのような計算を行っているのかも知っておく必要がありますが、気になる方は確認してみるといいでしょう。
transformでの予測
最後に、予測の部分を見てみましょう。
model.transform(test)
fitの結果はPipelineModelのインスタンスとなりますので、そちらのtrasformを見てみましょう
override def transform(dataset: Dataset[_]): DataFrame = { transformSchema(dataset.schema, logging = true) stages.foldLeft(dataset.toDF)((cur, transformer) => transformer.transform(cur)) }
pipeline内の各stageのtransformを順に呼び出しているだけとなっています。 Trasformerのtransformはすでに確認しているので、LogisticRegressionModelのtrasformを確認していましょう。
こちらはProbabilisticClassificationModelで定義されています。 こちらの処理も一部だけ見てみましょう。
if ($(rawPredictionCol).nonEmpty) { val predictRawUDF = udf { (features: Any) => predictRaw(features.asInstanceOf[FeaturesType]) } outputData = outputData.withColumn(getRawPredictionCol, predictRawUDF(col(getFeaturesCol))) numColsOutput += 1 }
行ごとの予測を行っています。 predictionRawは、LogisticRegressionで定義されています。
/** Margin (rawPrediction) for class label 1. For binary classification only. */ private val margin: Vector => Double = (features) => { BLAS.dot(features, _coefficients) + _intercept } override protected def predictRaw(features: Vector): Vector = { if (isMultinomial) { margins(features) } else { val m = margin(features) Vectors.dense(-m, m) } }
二値分類の場合、単純に計算をしているだけということがわかります。
ここまで処理で、exampleにおける、ざっくりとした全体像を眺めることができたかと思います。
終わりに
今回はexampleを起点にMLlibの実装を見てみました。
exampleをベースに追っていくので、そこで利用していない部分も含めての途中の該当するソースのクラス内すべては説明ていません。 また、説明を端折ったり、変数の初期化部分に関しては飛ばしたりなどもしています。
そのため、これだけでMLlibの全体像すべてを把握できるわけではなく、 自力で新しい手法を実装しようとした際にも共通化してある部分でどこがすでに用意されているものを利用できるのかなどを網羅的に知るにはそれらについても見ていく必要があります。
しかし、今回の内容程度のことを知っていれば、そういったことを行う際のとっかかりとしてどこを確認すればいいかの手がかりになるかと思います。